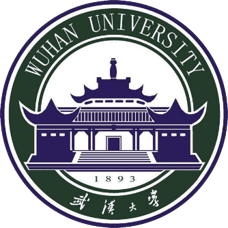
智能计算系统实验室(Intelligent Computing System Lab, ICSLab)主要研究领域为高性能计算,低功耗计算以及可扩展计算等分布式计算领域。我们的具体研究课题包含了云边计算系统,大数据平台,分布式深度学习架构,联邦学习,图计算,异构内存管理,低功耗算力平台等一系列智能计算系统。
程大钊,教授,博导,现任武汉大学计算机学院副院长。近期致力于研究面向人工智能负载的新型存内计算架构,具有丰富的计算机系统结构研究基础和经验。先后入选为湖北省“百人计划”特聘教授,“武汉英才”创新人才。同时,担任武汉大学计算机学院副院长,CCF分布式计算与系统专委会常务委员、国家重点研发计划和基金委重点项目首席科学家。2016-2020年在美国北卡大学计算机系担任Tenure Track助理教授,曾担任国家科技部、广东省和湖北省科技厅重点项目、美国和加拿大国家科学基金等评审专家。在权威计算机系统领域的期刊和会议上发表论文50篇并申请专利10多项,其中第一/通讯作者发表30余篇(含CCF A类20余篇)。主要从事存算一体化、分布式计算等方面的研究工作,先后主持了国家重点研发计划1项、国家自然科学基金委重点项目1项,美国NSF面上项目2项和其它省部级项目若干,共主持过科研项目经费约1700万元。同时应邀在多个国际国内学术组织中任职,担任4个学术期刊客座编委,5个国际会议的主席,28个国际会议的技术委员会委员。
• 电子邮箱: dcheng@whu.edu.cn
• 办公室: 计算机学院大楼A406
• Google Scholar
龚奕利,副教授,硕导。 1998年武汉大学计算机科学系本科毕业。2006年2月中科院计算所获得计算机体系结构专业博士学位,2006年至2007年在印第安纳大学Geoffrey Fox教授的Community Grids Lab从事博士后研究工作,2008年起至今在武汉大学计算机学院工作。2014年至2015年在密歇根大学安娜堡校区做为期一年的访问学者,合作导师为Sugih Jamin。 对各种分布式系统的研究都很感兴趣。目前,主要工作领域为HPC环境中的智能运维,分布式文件系统和区块链系统等。代表性论著(第一/通讯作者):Computer Journal 2016,HPCC 2015,ASAP 2017,ICPADS 2016,ICPP 2019等。
• 电子邮箱: yiligong@whu.edu.cn
• 办公室: 计算机学院大楼A515
胡创,副研究员,硕导。2019年香港理工大学计算机系博士毕业,2020年-2023年先后在香港理工大学继续担任博士后,研究助理教授。主要研究方向是边缘数据处理,联邦学习,分布式计算等。
• 电子邮箱: hchuchuang@gmail.com
• 办公室: 计算机学院大楼D502
车越之,博士后。2023年伊利诺伊理工大学计算机系博士毕业,后在美国圣母大学担任博士后。主要研究方向是计算机体系结构、安全与隐私保护等。
• 电子邮箱: cheyz2023@163.com
• 办公室: 计算机学院大楼E401
1. 高性能云计算
云计算将单个问题划分为多个部分,每个部分由不同的计算机解决,只要计算机联网,它们就可以相互通信进行大量数据交换以解决问题。我们进一步提出并开发了一种旨在提高多用户大数据集群的资源利用率的全新内存分布式计算框架。相关研究成果分别发表在
IEEE
TC'17,
IEEE
TPDS'18和
IEEE
INFOCOM'17。
2. 高性能边缘计算
边缘计算指的是在网络的边缘来处理数据,这样能够减少请求响应时间、提升电池续航能力、减少网络带宽同时保证数据的安全性和私密性。我们设计了基于任务重要性的多任务迁移学习任务优化系统,以提高在边缘支持迁移学习的效率。相关研究成果发表在
IEEE
Network'21,
IEEE
TPDS'20,
ICDCS'22。
3. 大数据平台
随着大数据时代的来临,数据量不断增长,传统单机的模式扩容困难且成本高昂,难以支撑业务发展,大数据平台的优化显得尤为重要。我们针对现有调度器在动态Hadoop大数据集群中的不足,提出一个基于动态资源感知的Hadoop
RDS调度器。相关研究成果分别发表在
IEEE
IPDPS'15、
IEEE
IPDPS'18,
IEEE
ICDCS'15和
IEEE
TPDS'17、
IEEE
TPDS'18上。
1. 低功耗边缘计算
在靠近用户的边缘端,注定是一个资源受限的(resource-constrained)环境,这时低功耗的运用对整个系统的性能提升就至关重要,甚至不考虑低功耗,这个系统就无法运行。实验室的桑乾龙同学正在研究移动端的动态调频技术,以便实现移动端的低功耗设计。
2. 低功耗云计算
节能型大数据集群的设计近年来引起了广泛关注。我们进一步提出了一种基于异构感知的任务分配方法E-Ant,旨在尽可能减少异构Hadoop集群的总能耗。该方法可以自适应地调度异构工作负载,而无需事先知道工作负载属性。研究成果发表在
IEEE
ICDCS'15上。后续成果发表在
IEEE
TPDS'18上。另有2篇关于绿色计算的论文分别发表在
IEEE
MASCOTS'13和
ACM
TAAS'15上。
3. 绿色数据中心
近年来在云计算产业发展浪潮下,建设绿色数据中心、实现节能减排成为了工业界关注的热门话题之一。在本研究中,我们提出了一种基于能源感知的绿色数据中心弹性资源配置方案。研究成果分别发表在
IEEE
TC'16和
IEEE
TPDS'18上。
1. 云边结合计算
边缘计算与云计算之间不是替代关系,而是互补协同关系。边缘计算与云计算需要通过紧密协同才能更好的满足各种需求场景的匹配,从而放大边缘计算和云计算的应用价值。我们提出一种边云结合的视频分析架构,显著降低视频分析延时。相关研究成果发表在
IEEE
Network'21,
IEEE
TPDS'20。
2. 分布式AI
为了提高预测的质量和使机器学习解决方案在更复杂的应用中可行,需要大量的训练数据,因此需要将机器学习工作量分散到多台机器上。我们针对性的设计了分布式GPU集群的调度器,该调度器能够有效率的调度并且合适地放置深度学习任务以减少他们的任务完成时间。相关研究成果发表在
ACM/IFIP/USENIX
2020上。另有3篇关于AI负载优化处理的论文分别发表在
IEEE
Big Data'19,
ACM
PPoPP'20和
IEEE
Cluster'20会议上。
3. 联邦学习/分析
联邦分析是由谷歌提出的一种分布式计算范式,它是在不公开边缘设备的本地数据的情况下共同执行分析任务。我们设计了一种主动防御局部模型毒化攻击的联邦异常分析分布式学习框架。相关研究成果发表在
ICDCS'19,
JSAC'22。
联邦学习的一个独特的特点是边缘设备属于个人,当面对大量的边缘设备时,集中式的模型聚合成为瓶颈,从根本上限制了系统的扩展性。我们设计了一个基于Spread的可扩展联邦系统:它采用了一种用于集群构建的自适应算法,在运行时调节集群间模型训练和集群内模型训练。相关成果发表在 ICPP'22。
期刊论文
[1] X Wei, ABMM Rahman, D Cheng, Y Wang: Joint Optimization across Timescales: Resource Placement and Task Dispatching in Edge Clouds. IEEE Transactions on Cloud Computing (TCC), 2021. (SCI一区)
View PDF[2] T Li; Z Qiu; D Cheng; W Wang; X Shi; Y Wang*: Privacy-Preserving Participant Grouping for Mobile Social Sensing over Edge Clouds. IEEE Transactions on Network Science and Engineering (TNSE), 2021.
View PDF[3] D Cheng; Y Wang; D Dai*: Dynamic Resource Provisioning for Iterative Workloads on Apache Spark. IEEE Transactions on Cloud Computing (TCC), 2021.(SCI一区)
View PDF[4] W Rang; D Yang; D Cheng*: Dependency-aware Tensor Scheduler for Industrial AI Applications. IEEE Industrial Electronics Magazine (IEM), 2021.(SCI一区)
View PDF[5] W Rang; D Yang; D Cheng*: Yu Wang; Data Life Aware Model Updating Strategy for Stream-based Online Deep Learning. IEEE Transactions on Parallel and Distributed Systems (TPDS), 2021.(CCF A类)
View PDF[6] D Yang, D Cheng*, W Rang, Y Wang: Joint Optimization of MapReduce Scheduling and Network Policy in Hierarchical Data Centers. IEEE Transactions on Cloud Computing (TCC), 2019.(SCI一区)
View PDF[7] D Cheng*, X Zhou, Y Xu, L Liu, C Jiang: Deadline-aware MapReduce job scheduling with dynamic resource availability. IEEE transactions on parallel and distributed systems (TPDS), 2018:30 (4), 814-826.(CCF A类)
View PDF[8] D Cheng*, X Zhou, Z Ding, Y Wang, M Ji: Heterogeneity aware workload management in distributed sustainable datacenters. IEEE Transactions on Parallel and Distributed Systems (TPDS),2018:30 (2), 375-387.(CCF A类)
View PDF[9] D Cheng*, X Zhou, Y Wang, C Jiang: Adaptive scheduling parallel jobs with dynamic batching in spark streaming. IEEE Transactions on Parallel and Distributed Systems (TPDS), 2018:29 (12), 2672-2685.(CCF A类)
View PDF[10] D Cheng*, X Zhou, P Lama, M Ji, C Jiang: Energy efficiency aware task assignment with dvfs in heterogeneous hadoop clusters. IEEE Transactions on Parallel and Distributed Systems (TPDS), 2017:29 (1), 70-82.(CCF A类)
View PDF[11] D Cheng, X Zhou*, P Lama, J Wu, C Jiang: Cross-platform resource scheduling for spark and mapreduce on yarn. IEEE Transactions on Computers (TC). 2017:66 (8), 1341-1353.(CCF A类)
View PDF[12] D Cheng, J Rao, Y Guo, C Jiang, X Zhou*: Improving performance of heterogeneous mapreduce clusters with adaptive task tuning. IEEE Transactions on Parallel and Distributed Systems (TPDS), 2016:28 (3), 774-786.(CCF A类)
View PDF[13] Y Guo, J Rao, D Cheng, X Zhou*: ishuffle: Improving hadoop performance with shuffle-on-write. IEEE transactions on parallel and distributed systems (TPDS), 2016:28 (6), 1649-1662.
View PDF[14] D Cheng, J Rao, C Jiang, X Zhou*: Elastic power-aware resource provisioning of heterogeneous workloads in self-sustainable datacenters. IEEE Transactions on Computers (TC). 2015:65 (2), 508-521.(CCF A类)
View PDF[15] D Cheng, Y Guo, C Jiang, X Zhou*: Self-tuning batching with dvfs for performance improvement and energy efficiency in internet servers. ACM Transactions on Autonomous and Adaptive Systems (TAAS), 2015:10 (1), 1-32.
View PDF[16] Yili Gong; Chuang Hu; Yanyan Xu; Wenjie Wang; A distributed file system with variable si zed objects for enhanced random writes, Computer Journal, 2016, 59(10): 1536-1550
View PDF[17] Chuang Hu, Rui Lu, and Dan Wang, “FEVA: A FEderated Video Analytics Architecture for Networked Smart Cameras”, IEEE Network, Volume 35, Issue 6, Pages 163-170, December, 2021. [SCI 一区]
View PDF[18] Siping Shi, Chuang Hu, Dan Wang, and Yifei Zhu, and Zhu Han, “Federated Anomaly Analytics for Local Model Poisoning Attack”, IEEE Journal on Selected Areas in Communications (JSAC), Volume 40, Issue 2, Pages 596-610, February, 2022. [CCF-A, SCI 一区]
View PDF[19] Chuang Hu, Wei Bao, Dan Wang, Yi Qian, Muqiao Zheng and Shi Wang, “sTube+: An IoT Communication Sharing Architecture for Smart After-sales Maintenance in Buildings”, ACM Transactions on Sensor Networks (TOSN), Volume 14, Issue 3-4, Pages 1-29, December, 2018. [CCF-B,SCI 三区]
View PDF[20] Qiong Chen, Zimu Zheng, Chuang Hu, Dan Wang, and Fangming Liu,“On-Edge Multi-Task Transfer Learning: Model and Practice With Data-Driven Task Allocation”, IEEE Transactions on Parallel and Distributed Systems (TPDS), Volume 31, Number 6, Pages 1357-1371, June, 2020. [CCF-A, SCI 二区]
View PDF[21] Dan Wang, Wei Bao, Chuang Hu, Yi Qian, Muqiao Zheng and Shi Wang, “sTube: An Architecture for IoT Communication Sharing”, IEEE Wireless Communications Magazine, Volume 56 , Issue 7, Pages 96-101, July, 2018. [SCI 一区]
View PDF[22] Yili Gong, Chuang Hu, Yanyan Xu, et al., “A Distributed File System with Variable Sized Objects for Enhanced Random Writes”, The Computer Journal, Volume 59, Number 10, Pages 1536-1550, 2016. [CCF-B, SCI 四区]
View PDF[23] 魏森;周浩然;程大钊*;胡创,基于混合内存的Apache Spark缓存系统实现与优化[J]. 计算机科学, 2023.
View PDF[24] H Liang, Q Sang, C Hu, D Cheng*, X Zhou, D Wang, W Bao, Y Wang. DNN Surgery: Accelerating DNN Inference on the Edge through Layer Partitioning[J]. IEEE Transactions on Cloud Computing, 2023. (SCI二区)
View PDF[25] Zheng Zhang, Yaqi Xia, Hulin Wang, Donglin Yang, Chuang Hu;Xiaobo Zhou, Dazhao Cheng: MPMoE: Memory Efficient MoE for Pre-trained Models with Adaptive Pipeline Parallelism
View PDF会议论文
[1] W Rang, D Yang, Z Li, D Cheng: Scalable Data Management on Hybrid Memory System for Deep Neural Network Applications. 2021 IEEE International Conference on Big Data (Big Data), 1470-1480
View PDF[2] K Suo, J Son, D Cheng, W Chen, S Baidya: Tackling Cold Start of Serverless Applications by Efficient and Adaptive Container Runtime Reusing. (CLUSTER 2021): 433-443
View PDF[3] W Rang, D Yang, D Cheng: A Shared Memory Cache Layer across Multiple Executors in Apache Spark.2020 IEEE International Conference on Big Data (Big Data), 477-482.
View PDF[4] D Yang, W Rang, D Cheng: Mitigating Stragglers in the Decentralized Training on Heterogeneous Clusters.Proceedings of the 21st International Middleware Conference (Middleware), 386-399.
View PDF[5] W Rang, D Yang, D Cheng*, K Suo, W Chen: Data Life Aware Model Updating Strategy for Stream-based Online Deep Learning.2020 IEEE International Conference on Cluster Computing (CLUSTER), 392-398.
View PDF[6] K Suo, Y Shi, X Xu, D Cheng, W Chen:Tackling Cold Start in Serverless Computing with Container Runtime Reusing.Proceedings of the Workshop on Network Application Integration/CoDesign, 54-55.
View PDF[7] D Yang, D Cheng: Efficient gpu memory management for nonlinear dnns. Proceedings of the 29th International Symposium on High-Performance Parallel and Distributed Computing (HPDC), 185-196.
View PDF[8] J Tian, S Di, C Zhang, X Liang, S Jin, D Cheng, D Tao*, F Cappello: Wavesz: A hardware-algorithm co-design of efficient lossy compression for scientific data. Proceedings of the 25th ACM SIGPLAN Symposium on Principles and Practice of Parallel Programming (PPoPP), 74-88.
View PDF[9] D Yang, W Rang, D Cheng, Y Wang, J Tian, D Tao:Elastic Executor Provisioning for Iterative Workloads on Apache Spark. 2019 IEEE International Conference on Big Data (Big Data), 413-422.
View PDF[10] TBG Perez, X Zhou, D Cheng: Reference-distance eviction and prefetching for cache management in spark. Proceedings of the 47th International Conference on Parallel Processing (ICPP), 1-10 12.
View PDF[11] D Yang, W Rang, D Cheng: Joint optimization of mapreduce scheduling and network policy in hierarchical clouds. Proceedings of the 47th International Conference on Parallel Processing (ICPP), 1-10.
View PDF[12] P Lama, S Wang, X Zhou, D Cheng: Performance isolation of data-intensive scale-out applications in a multi-tenant cloud. 2018 IEEE International Parallel and Distributed Processing Symposium (IPDPS), 85-94.
View PDF[13] D Cheng, Y Chen, X Zhou, D Gmach, D Milojicic: Adaptive scheduling of parallel jobs in spark streaming. IEEE INFOCOM 2017-IEEE Conference on Computer Communicationsc(INFOCOM), 1-9.(CCF A类)
View PDF[14] D Cheng, P Lama, C Jiang, X Zhou: Towards energy efficiency in heterogeneous hadoop clusters by adaptive task assignment. 2015 IEEE 35th International Conference on Distributed Computing Systems (ICDCS). 359-368.
View PDF[15] D Cheng, J Rao, C Jiang, X Zhou: Resource and deadline-aware job scheduling in dynamic hadoop clusters. 2015 IEEE International Parallel and Distributed Processing Symposium (IPDPS), 956-965.
View PDF[16] Y Guo, J Rao, D Cheng, C Jiang, CZ Xu, X Zhou: Storeapp: A shared storage appliance for efficient and scalable virtualized hadoop clusters. 2015 IEEE Conference on Computer Communications (INFOCOM), 594-602.
View PDF[17] D Cheng, J Rao, Y Guo, X Zhou: Improving mapreduce performance in heterogeneous environments with adaptive task tuning. Proceedings of the 15th International Middleware Conference (Middleware), 97-108.
View PDF[18] D Cheng, C Jiang, X Zhou: Heterogeneity-aware workload placement and migration in distributed sustainable datacenters. 2014 IEEE 28th International Parallel and Distributed Processing Symposium (IPDPS), 307-316.
View PDF[19] D Cheng, Y Guo, X Zhou: Self-tuning batching with dvfs for improving performance and energy efficiency in servers. 2013 IEEE 21st International Symposium on Modelling, Analysis and Simulation of Computer and Telecommunication Systems (MASCOTS),40-49.
View PDF[20] Yili Gong; Yanyan Xu; Yingchun Lei; Wenjie Wang ; VarFS: A variable-sized objects based d istributed file system, 2015 IEEE 17th International Conference on High Performance Computing and Communications, New York, NY, United states, 2015-08-24至2015-08-26
View PDF[21] Yili Gong; Jia Tang; Wenhai Li; Zihui Ye ; Massive spatial query on the Kepler architectu re, 2017 IEEE 28th International Conference on Application-specific Systems, Architectures and Pr ocessors (ASAP), Seattle, WA, USA, 2017-7-10至2017-7-12
View PDF[22] Yili Gong; Chuang Hu; Wentao Ma; Wenjie Wang ; CC-Paxos: Integrating Consistency and Reli ability in Wide-Area Storage Systems, 22nd IEEE International Conference on Parallel and Distribu ted Systems (ICPADS 2016), Wuhan, China, 2016-12-13至2016-12-16
View PDF[23] Caixin Gong; Shuibing He; Yili Gong; Yingchun Lei ; On Integration of Appends and Merges in Log-Structured Merge Trees, Proceedings of the 48th International Conference on Parallel Proce ssing - ICPP 2019, Kyoto, Japan, 2019-8-5至2019-8-8
View PDF[24] Chuang Hu, Wei Bao, Dan Wang, and Fengming Liu, “Dynamic Adaptive DNN Surgery for Inference Acceleration on the Edge”, Proceedings of the 38th IEEE International Conference on Computer Communication (INFOCOM’19), Paris, France, April 29 - May 2, 2019. [CCF-A]
View PDF[25] Chuang Hu, Wei Bao, and Dan Wang, “IoT Communication Sharing: Scenarios, Algorithms and Implementation”, Proceedings of the 37th IEEE International Conference on Computer Communication (INFOCOM’18), Honolulu, HI, October 24-28, 2018. [CCF-A]
View PDF[26] Chuang Hu, Huanghuang Liang, Xiaoming Han, Boan Liu, Dazhao Cheng, and Dan Wang, “Spread: Decentralized Model Aggregation for Scalable Federated Learning”, 51st International Conference on Parallel Processing (ICPP’22), Bordeaux, France, August 29- September 1, 2022. [CCF-B]
[27] Rui Lu, Chuang Hu*, Dan Wang, and Jin Zhang, “Gemini: a Real-time Video Analytics System with Dual Computing Resource Control”, The Seventh ACM/IEEE Symposium on Edge Computing (SEC’22), Seattle, WA, December 5-8, 2022.
[28] Siping Shi, Chuang Hu, Dan Wang, and Yifei Zhu, and Zhu Han, “Distributionally Robust Federated Learning for Differentially Private Data”, 42nd IEEE International Conference on Distributed Computing Systems (ICDCS’22), Bologna, Italy, July 10-13, 2022. [CCF-B]
[29] Chuang Hu, Wei Bao, Dan Wang, Yi Qian, Muqiao Zheng and Shi Wang, “sTube+: An IoT Communication Sharing Architecture for Smart After-sales Maintenance in Buildings”, Proceedings of the 4th ACM International Conference on Systems for Energy-Efficient Build Environments (BuildSys’17), Delft, The Netherlands, November 8-9, 2017.
View PDF[30] Junkun Peng, Qing Li, Xiaoteng Ma, Yong Jiang, Yutao Dong, Chuang Hu and Meng Chen, “MagNet: Cooperative Edge Caching by Automatic Content Congregating”, Proceedings of the ACMWeb Conference (WWW’22), accepted, 2022. [CCF-A]
View PDF[31] Qiong Chen, Zimu Zheng, Chuang Hu, Dan Wang, and Fangming Liu, “Data-driven Task Allocation for Multi-task Transfer Learning on the Edge”, Proceedings ofthe IEEE International Conference on Distributed Computing Systems (ICDCS’19), Dallas, Texas, July 7-9, 2019. [CCF-B]
View PDF[32] Zimu Zheng, Chuang Hu, and Dan Wang, “Time-aware Chiller Sequencing Control with Data-driven Chiller Performance Profiling (Poster)”, Proceedings of the 4th ACM International Conference on Systems for Energy-Efficient Build Environments (BuildSys’17), Delft, The Netherlands, November 8-9, 2017.
View PDF[33] Yili Gong, Chuang Hu , Wentao Ma, et al., “CC-Paxos: Integrating Consistency and Reliability in Wide-Area Storage Systems”, in Proceedings of IEEE International Conference on Parallel and Distributed Systems (ICPADS’16) , Wuhan, China, December 2016. [CCF-C]
View PDF[34] Z Zhang, D Yang, Y Xia, L Ding, D Tao, X Zhou, D Cheng*.MPipeMoE: Memory Efficient MoE for Pre-trained Models with Adaptive Pipeline Parallelism. 37th IEEE International Parallel &Distributed Processing Symposium (IPDPS), 1-11.
View PDF[35] A. Al Raqibul Islam, D. Dai, D. Cheng, VCSR: Mutable CSR Graph Format Using Vertex-Centric Packed Memory Array. 22nd IEEE International Symposium on Cluster, Cloud and Internet Computing (CCGrid),71-80.
View PDF[36] C Hu, H Liang, B Liu, X Han, D Wang, D Cheng*, Spread: Decentralized Model Aggregation for Scalable Federated Learning, 51st International Conference on Parallel Processing ( ICPP), 1-12.
View PDF• 实验室配备了16台集群服务器和1台内存服务器,服务器之间通过千兆网络连接。主要用于实验室学生共享使用进行实验或者进行集群级别实验。
• 实验室在计算机学院机房内配置了刀片机服务器,主要用于虚拟化实验和深度学习计算。A100服务器的GPU卡之间通过NVLink连接。
• 除了实验室配备的资源外,还可以借助武汉大学超算中心的资源进行实验研究。
• 鲲鹏服务器:搭配 kunpeng920 * 2 国产Arm CPU, 32GB*6 内存, 480G SSD + 4T HDD存储
• 该项目致力于研究寻Arm服务器下的虚拟化,通过对KVM虚拟化技术深入研究,实现Arm架构下的一套高效的虚拟机管理系统,涉及到的领域包括虚拟化技术、分布式存储技术、分布式通信等。
• 智能无人车:配备4台智能无人车,搭载深度相机与激光雷达
• 智能无人车搭载了镭神激光雷达和Astra Pro深度相机,借助激光雷达和深度相机建立的VSLAM框架可以实现近、中距离的同步定位与地图构建。智能无人车支持RTAB视觉SLAM,支持激光雷达建图导航,支持声源定位与语音导航,通过对智能车硬件的研究和对模型的优化与调整,可以在智能车的平台上设计实现深度视觉识别、激光雷达建图、自主导航避障等应用。
• 拥有6台智能无人车(履带式*1,轮式*1,微型无人车*4),搭载深度相机、激光雷达与边缘计算套件。
• 本实验室构建了一套自主可控的无人集群系统,依托异构无人车底盘与低功耗智能计算单元,开发SLAM算法、环境语义理解与表征模型、运动控制策略、有限带宽信息传输技术,形成涵盖 “感知-理解-表征”全链路的多智能体语义建图软硬件平台。集群系统支持声源定位与语音导航、广域场景协同探索与环境认知、自主巡视与避障等应用。
• OnePlus9 Pro:配备4台骁龙888 12GB一加9Pro
• Raspberry Pi 4 Computer:配备20块1GB RAM树莓派
• 边缘计算项目致力于研究移动设备性能和功耗的平衡和优化,通过对内核中异构CPU调度和调频技术的研究,提出基于人工智能算法的性能感知联合调度调频算法,实现保证用户性能的前提下手机系统功耗大幅度下降。
实验室邀请到澳门大学的王也教授作学术报告:How Crises Impact Stablecopn Trading Behaviors?
实验室邀请到斯图加特大学的Michael M. Resch教授作学术报告:Simulation on Supercomputers
程大钊教授受邀在第十一届全国软件工程研究生教育研讨会做特邀报告。报告分析了新形势下软件工程专业研究生培养面临的挑战、应对策略和实践举措及成效。
实验室硕士生屠天宇在“KubeEdge Rising Star Award 2023 (第1届)”中获得新星贡献奖。
实验室邀请到新加坡国立大学的陈瑶教授作学术报告:From Applications to Efficient Architectures on FPGAs
实验室邀请到悉尼大学的包魏教授作学术报告:Towards Efficient Distributed Machine Learning: A Joint Algorithm and System Approach
实验室邀请到澳门大学的王也教授、周晟炜博士作学术报告
实验室邀请到德克萨斯大学阿灵顿分校的Jia Rao教授作学术报告:“Architecture and Software Optimizations for Future Memory Technology”
实验室邀请到美国英伟达公司高级工程师杨东林博士作三场学术报告:“Deep Learning Systems: Design and Implementation”
Redundancy-Free High-Performance Dynamic GNN Training with Hierarchical Pipeline Parallelism(best paper candidate)
YaqiXia, Zheng Zhang, Hulin Wang, Donglin Yang, Xiaobo Zhou, Dazhao Cheng
实验室博士生何智力在2023年武汉大学乒乓球协会精英杯乒乓球赛中获得男子单打第三名。
MPipeMoE: Memory Efficient MoE for Pre-trained Models with Adaptive Pipeline Parallelism
Zheng Zhang, Donglin Yang, Yaqi Xia, Liang Ding, Dacheng Tao, Xiaobo Zhou, Dazhao Cheng
实验室首篇中文论文:《基于混合内存的Apache Spark缓存系统实现与优化》魏森、周浩然、胡创、程大钊 获评计算机学会分布式系统专委会年会最佳论文(2/69)。
实验室博士生何智力在武汉大学乒乓球协会“新生杯”乒乓球比赛男子单打项目中获得季军。
实验室邀请到UTSA的刘天义博士作题目为“Enabling 3D Applications in Public Cloud”的学术报告。
首先实验室有着充足的经费,能够为你排除科研路上一切外在障碍。其次我们能够提供丰富的科研课题,尽早发表文章减轻毕业压力。同时我们有着优秀的老师能够耐心指导你进行研究与写作。欢迎你来到ICSLab,为实验室的科研建设添砖加瓦,更为自己的前程铺平道路。
从前的好友各赴前程,初入武大难免担忧。
当下的科研踌躇满志,你却不知何从下手。
未来的选择丰富多彩,你该如何从心所欲。
来到ICSLab,你会遇到帅气随和的导师,热心上进的师兄师姐。无论是科研或是生活,总有一个人能够为你答疑解惑,帮你跨入研究生的门槛。我们有着丰富多样的科研方向可以选择,也有着五花八门的横向课题用于实践,更有着优渥的津贴补助,欢迎每一个对实验室感兴趣的同学投递简历!
在这里,无论你是想发表文章,或者是进行科研竞赛,我们都能提供充分的指导与协助。实验室的监督也能帮你养成更好的学习习惯,从而不虚度本科时光。更为重要的是,ICSLab能够为你提供毕设指导,帮助你进行选题以及论文撰写,为自己本科生涯画上一个理想的句号。